This week, I have attended the 16th International Conference on Advanced Data Mining and Applications (ADMA 2021) conference, which is held online due to the COVID pandemic.
What is ADMA ?
ADMA is a medium-scale conference that focus on data science and its applications (hence its name). The ADMA conference is generally held in China but was twice in Australia and once in Singapore. I participated to this conference several times. If you want to read my report about previous ADMA conferences, you can click here: ADMA 2019, ADMA 2018, ADMA 2013 and ADMA 2014.
This time, the conference was called ADMA 2021, although it is held from the 2nd to 4th February 2022. The reason why the conference is held in 2022 is that it was postponed due to the COVID-19 pandemic. ADMA 2021 was co-located with the australasian artificial intelligence conference (AJCAI 2021), which is a national conference about AI.
Proceedings
The proceedings are published by Springer in the Lecture Notes in Artificial Intelligence series as two volumes. The proceedings contain 61 papers, among which 26 were presented orally at the conference while the remaining were presented as posters.
The papers were selected from 116 paper submissions, which means an overall acceptance rate of 61 / 116 = 52 % and 22 % for the papers presented orally.

Schedule
The ADMA conference was held on three days. There was three keynote talks, two panels, six invited talks, two hours for poster sessions and some regular paper sessions. The schedule is below.
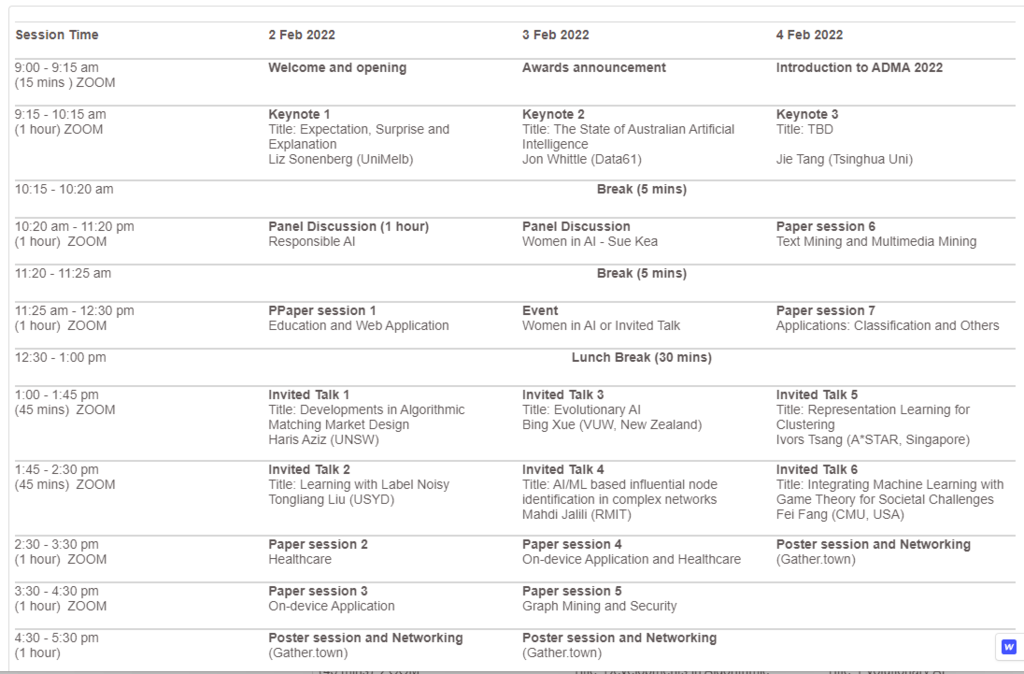
The conference was held according to the Australian time zone, which means that I had to wake up at 6 PM in China to see the first events.
A virtual conference
The ADMA conference was hosted on the Zoom platform for viewing the presentations and another platform called GatherTown for social interactions. The Gathertown platform is used by several conferences. Using this platform, each attendee can create an avatar and to explore a 2D world. Then, when you go closer to the avatar of another person, you can have a discussion via webcam and microphone with that person. This allows to recreate a little bit the atmosphere of a real conference. Here are a few screenshots of this virtual environment:


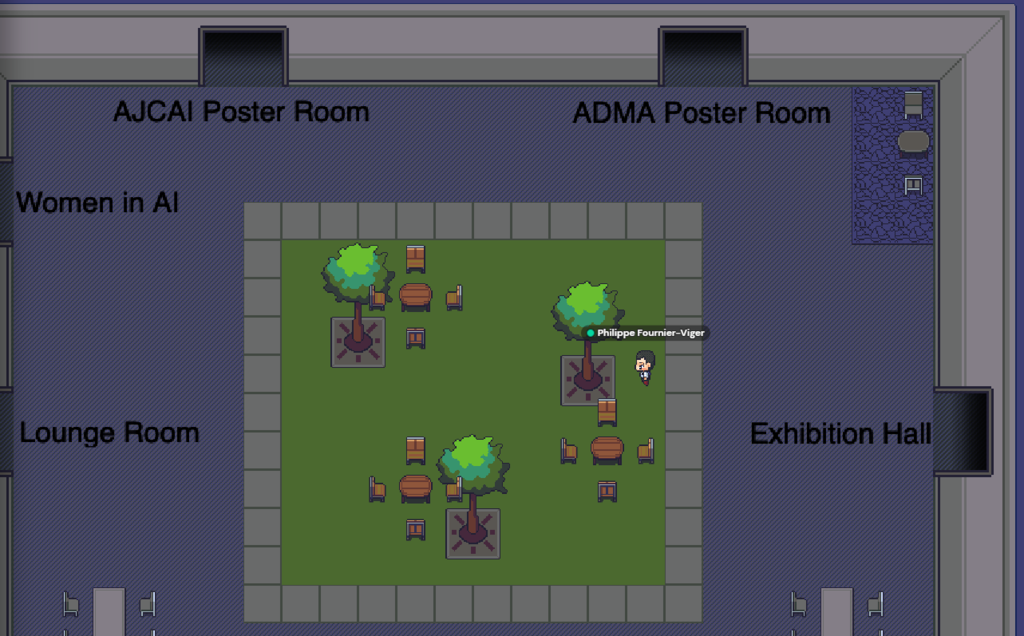

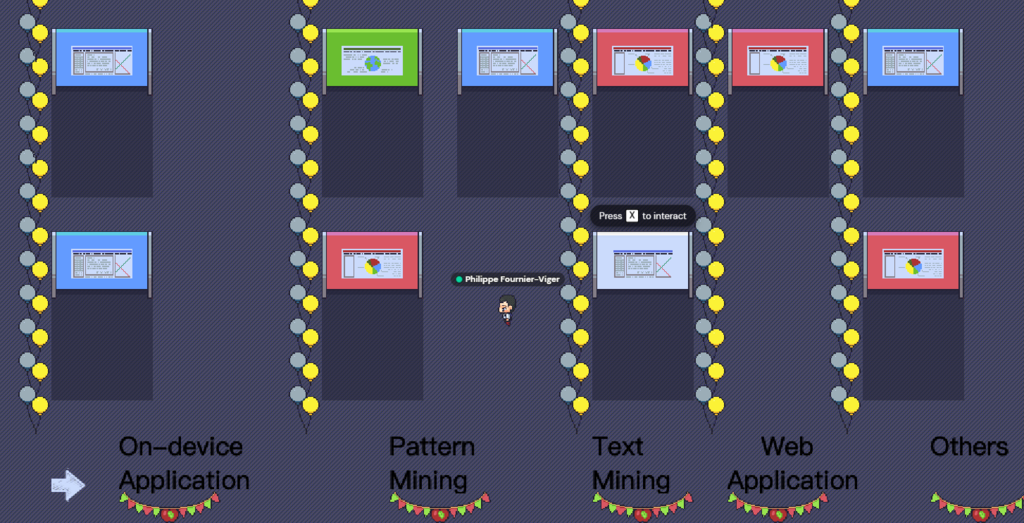
Day 1 – Panel on Responsable AI
On the first day, there was a panel on responsable AI with 4 invited panelists. The discussion was on topics such as how to improve the brand of Australia for AI, the need on more funding for Responsabble AI in Australia, AI regulations, AI ethics, deepfakes, etc.
Day 1 – Paper sessions
On the first day, there was also some paper sessions. There was several topics such as personalized question recommendation, cheating detection, a paper about a new dataset, and medical applications.
Day 1 – Poster sessions
The poster session was held in Gather Town. During the poster session, I have stayed mostly beside my poster in case some people would come to talk with me. It works as follows. If some persons approach your poster, then it starts a webcam discussion with them. There was over 60 persons online at that time. I have discussed with maybe 4 or 5. Here are a few screenshots from the poster session:
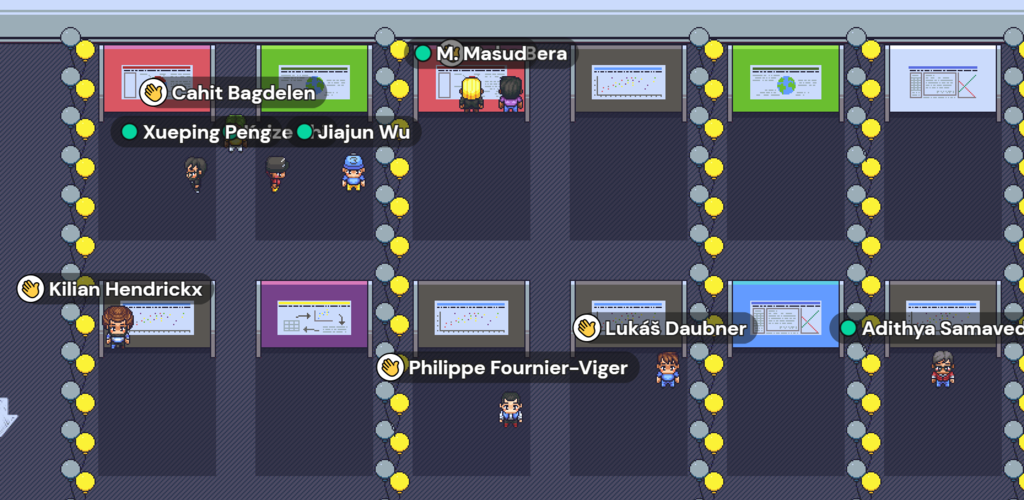

Globally, this idea of using GatherTown is interesting. It allows to make some social interactions, which otherwise would be lacking for a virtual conference. However, some thing that I think could be improved about poster sessions in GatherTown is that there is no index or search function to find a poster. Thus, to search for a poster we must go around the room to try to find it, which takes time. Also another area for improvement is that when showing a poster to another attendee, that person cannot see your mouse cursor. That is something that GatherTown developers could improve.
Pattern mining papers
As readers of this blog know, I am interested by pattern mining research. So here, I have made a list of the main pattern mining papers presented at the conference:
- 1- “SMIM framework to generalize high-utility itemset mining”: a framework for high utility itemset mining.
- 2- “TKQ: Top-K Quantitative High Utility Itemset Mining: a new algorithm for top-k high utility quantitative itemset mining
- 3- “OPECUR: An enhanced clustering-based model for discovering unexpected rules”
- 4- “Extracting High Profit Sequential Feature Groups of Products using High Utility Sequential Pattern Mining”
- 5- “Game Achievement Analysis: Process Mining Approach”
- 6- “Tourists Profiling by Interest Analysis”
It is interesting to see that three of this papers are related to high utility pattern mining, a popular research direction in pattern mining. The last paper is related to process mining, which is also a popular topic about the application of pattern mining and data mining to analyze business processes.
Award ceremony
I missed the award ceremony because it started very early (6:00 AM) in my time zone (China) so I will not report the details about awards but I got the news that I received this award afterward:
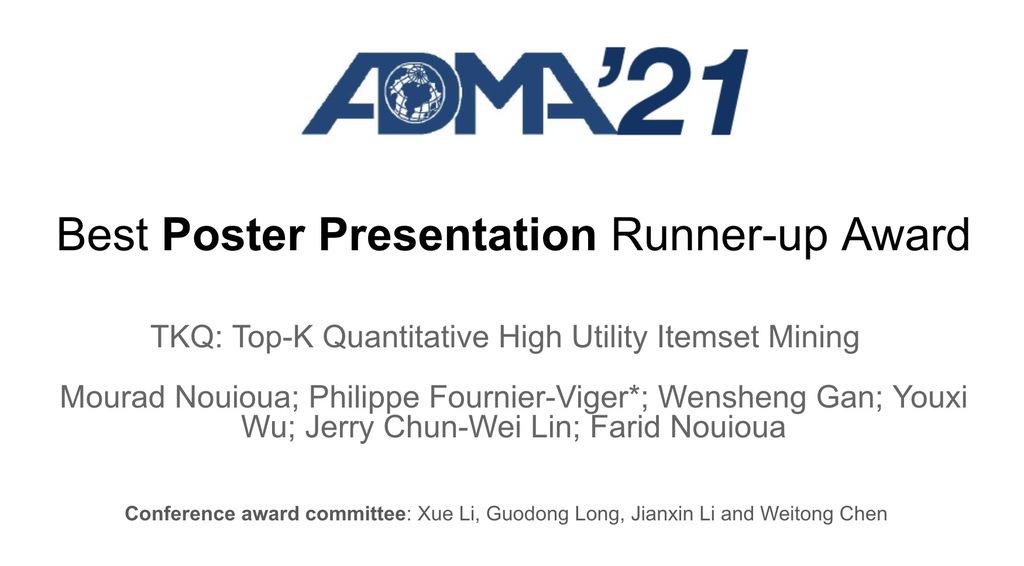
Next ADMA conference (ADMA 2022)
The next ADMA conference will be called ADMA 2022 and be in Brisbane, Australia, probably around December.

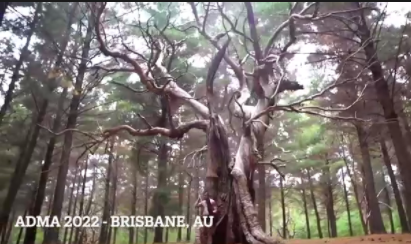
Conclusion
Overall, ADMA 2021 was a good conference. That is all for today!
—
Philippe Fournier-Viger is a full professor, working in China, and founder of the SPMF open-source data mining library.