Today, I want to talk a little bit about the next version of SPMF that is coming very soon. Here is some highlights of the upcoming features:
1) A Memory Viewer to help monitor the performance of algorithms in real-time:
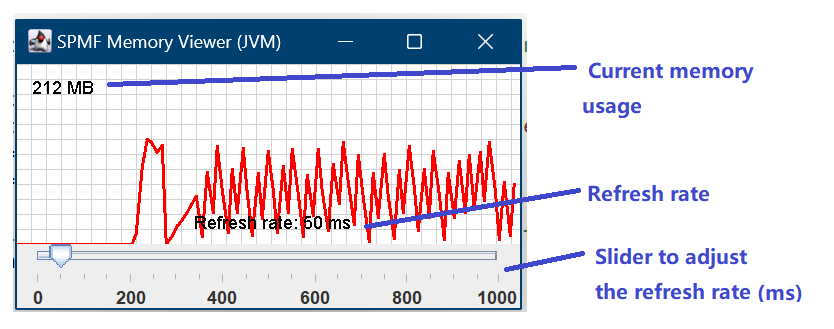
Also, the popular MemoryLogger class of SPMF is also improved to provide the option of saving all recorded memory values to a file when it is set in recording mode and a file path is provided. This is done using two new methods “startRecordingMode” and “stopRecordingMode”. The MemoryLogger will then write the memory usage values to a file every time that an algorithm calls the checkMemory method. You can stop the recording mode by calling the stopRecordingMode method.
2) A tool to generate cluster datasets using different data distributions such as Normal and Uniform distribution. Here some screenshots of it:
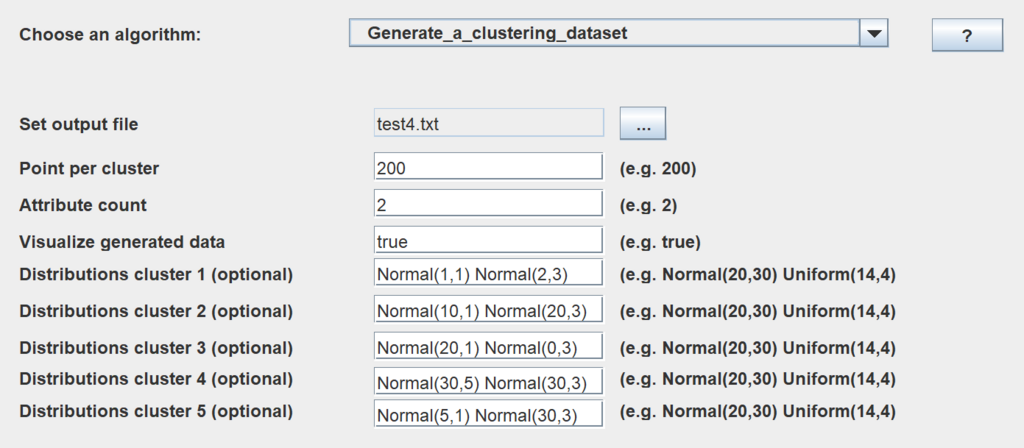
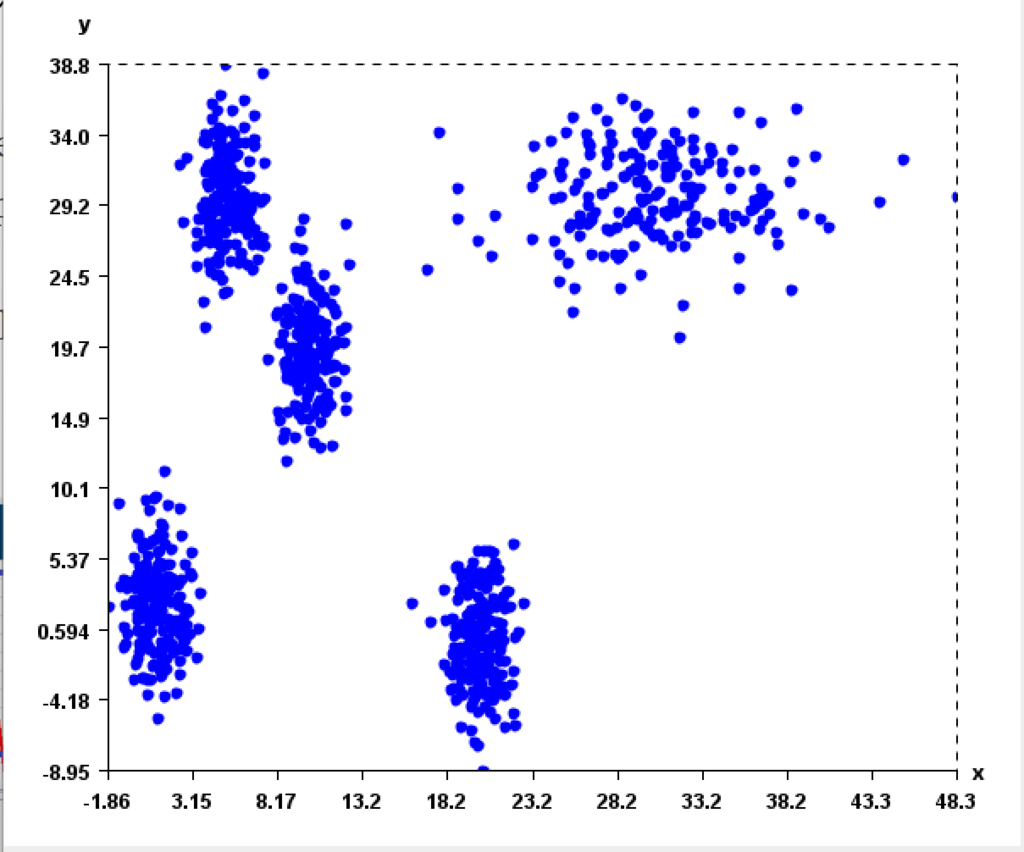
3) A simple tool to visualize transactions datasets. This tool is simple but can be useful for quickly exploring a datasets and see the content. It provides various information. This is an early version. More features will be considered.
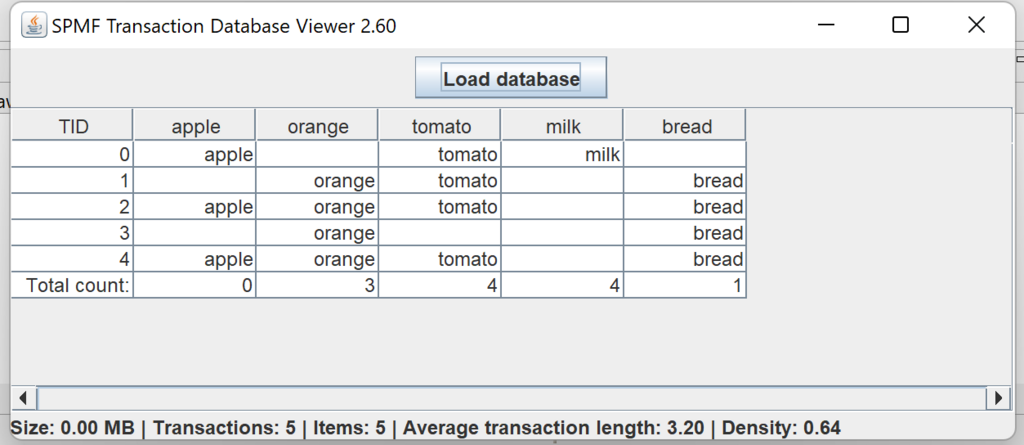
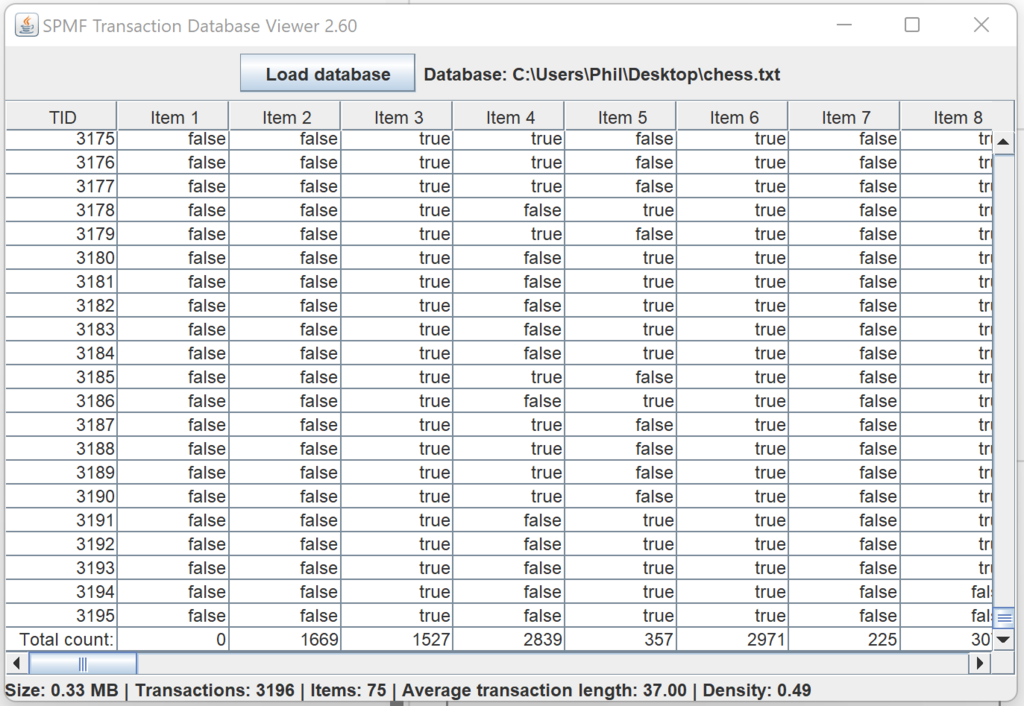
The tool has two visualization features, to viewthe frequency distribution of transaction according to their lengths, as well as the frequency distribution of items according to their support:
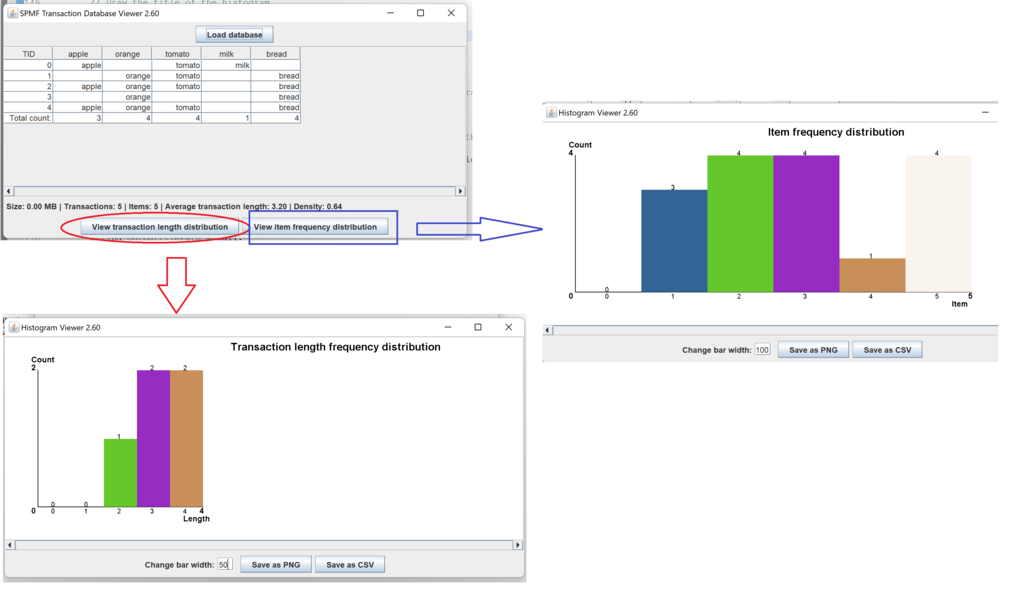
4) A simple tool to visualize sequence datasets. This is similar to the above tool but for sequence datasets.
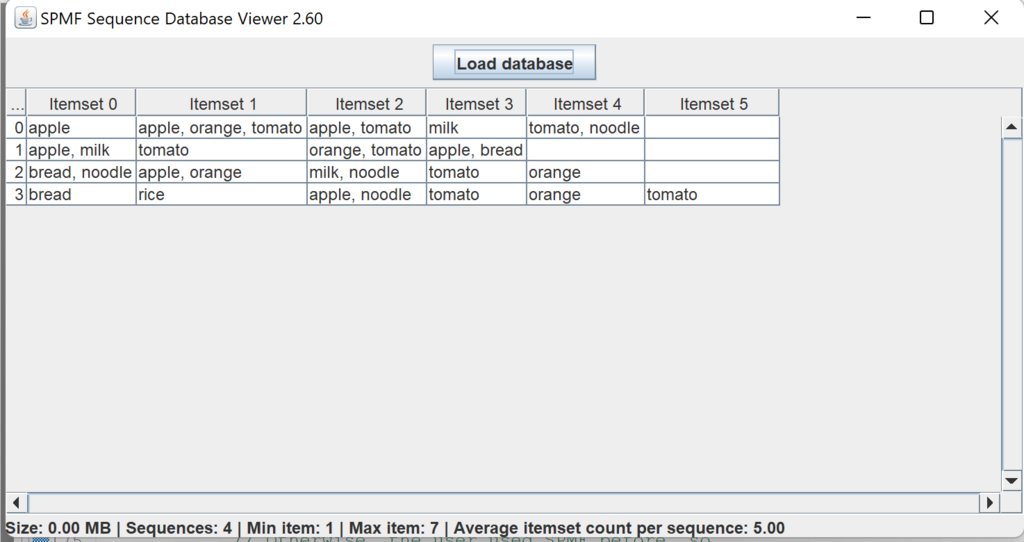
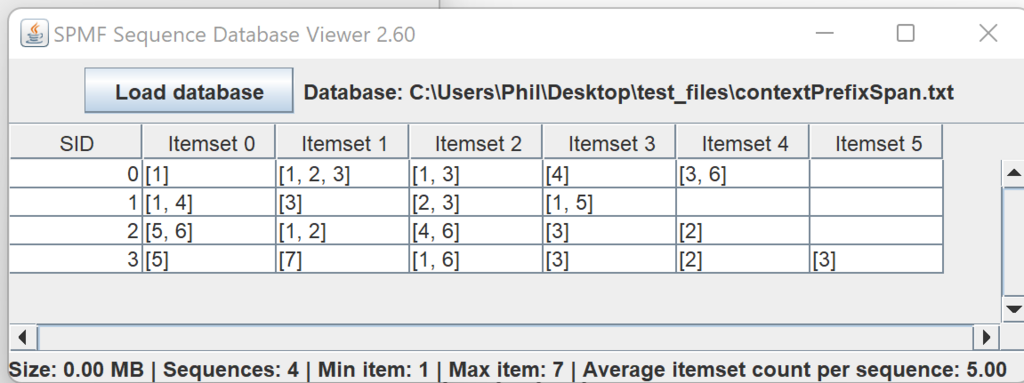
5) A new tool to visualize the frequency distribution of patterns found by an algorithm. To use this feature, when running an algorithm select the “Pattern viewer” for opening the output file. Then, select the support #SUP and click “View”. This will open a new window that will display the frequency distribution of support values, as show below. This feature also works with other measures besides the support such as the confidence, and utility.
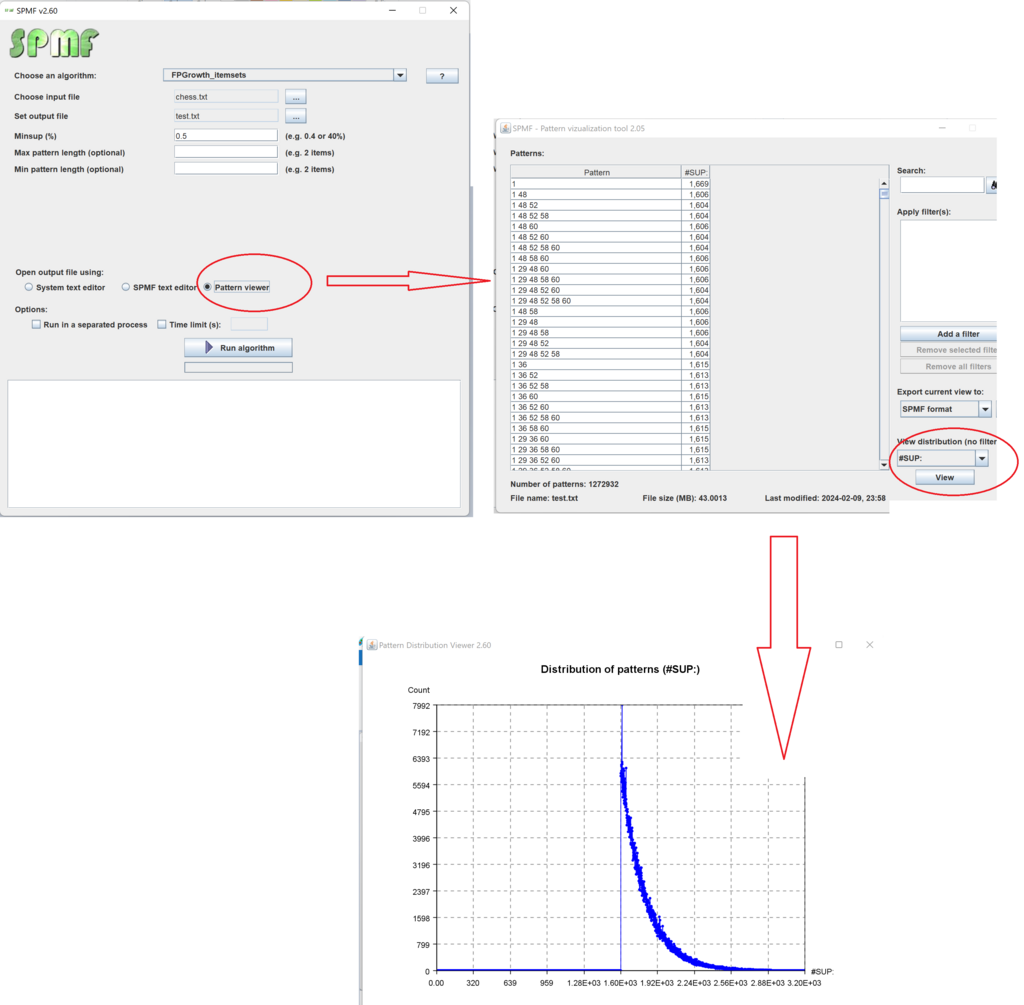
6) A tool to compute statistics about graph database files in SPMF format. This is a feature that was missing in previous version of SPMF but is actually useful when working with graph datasets.

7) Several new data mining algorithm implementations. Of course, several algorithms for data mining will be added. Some that are ready are FastTIRP, VertTIRP, Krimp, and SLIM. Others are under integration.
8) A new set of highly efficient data structures implemented using primitive types to further improve the performance of data mining algorithms by replacing standard collection classes from Java. Some of those are visible in the picture below. Using those structure can improve the performance of algorithm implementations. It actually took weeks of work to develop these classes and make it compatible with comparators and other expected features of collections in the Java language.
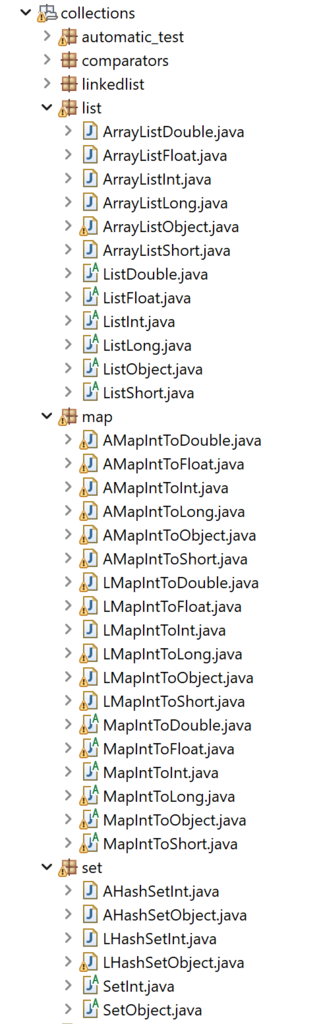
Conclusion
This is just to give you an overview about the upcoming version of SPMF. I hope to release it in the next week or two. By the way, if anyone has implemented some algorithms and would them to be included in SPMF, please send me an e-mail at philfv AT qq DOT com.
—
Philippe Fournier-Viger is a distinguished professor working in China and founder of the SPMF open source data mining software.