A month ago, I had some big problems with my website hosted by 1and1 ionos. The database was seemingly reverted to three back and I lost all the posts from the last three years! This week, I got more problems with 1and1 (also known as 1&1 IONOS). I have several days of downtime due to their database server going offline. I am am not happy with the service. Here is the story.
October 1st 2020. Recently, I noticed that my data mining forum (https://forum2.philippe-fournier-viger.com/ ) hosted on 1and1 Ionos went offline due to a technical problem. When I connected to the website, it has shown this error:

First, I thought that the problem was something going wrong on my website or that I may have been hacked. So, I used the 1and1 Ionos control panel to reset the password of the database and I also downloaded the sample PHP script from them to connect to the database to ensure that it was not a problem of my website:

But that script also failed to connect. Thus, I clicked on the Ionos control panel to see the database directly through PHPMyAdmin:
Then, I got that error indicating that the database is offline:
Here, the message is in Chinese, but basically it says:
mysqli_real_connect(): (HY000/1045): Access denied for user ‘dbo276830812’@’10.72.2.8’ (using password: YES)
PhpMyAdmin tried to connect to the MySQL server, but the server refused to connect. You should check the host, user name, and password in the configuration file and make sure that the information is consistent with the information given by the MySQL server administrator.”
So obviously, since I cannot even connect to the database through the 1and1 control pannel, it is a problem on the side of 1and1 Ionos. And this is why my website has gone offline.
October 2nd: I sent an e-mail to 1and1 Ionos to inform them about the problem through the customer support e-mail at 1:09 AM on October 2nd (Beijing Time). Here is their answer promising that I would be answered whithin 48 hours:
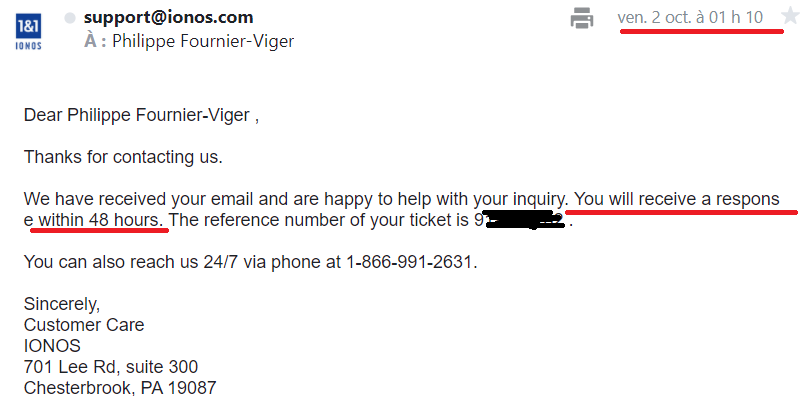
October 4th: I still did not receive any news from 1and1 Ionos and the website is still down because of the database being unavailable. So on the evening, I contacted the 1and1 IONOS customer service again through the live chat to see what is going on and the representative told me that my request is in the customer support system and has been escalated. He also told me that they are sorry for the inconvenience, and told me to wait a bit and he would check something in the system. Then for some reason (poor internet connection?), the customer support live chat window closed. So I decided to wait until tomorrow.
October 5th: About 10 hours later, it is already more than four days that the website is down due to this technical problem… Finally, the problem was fixed and got some answer from the customer service:
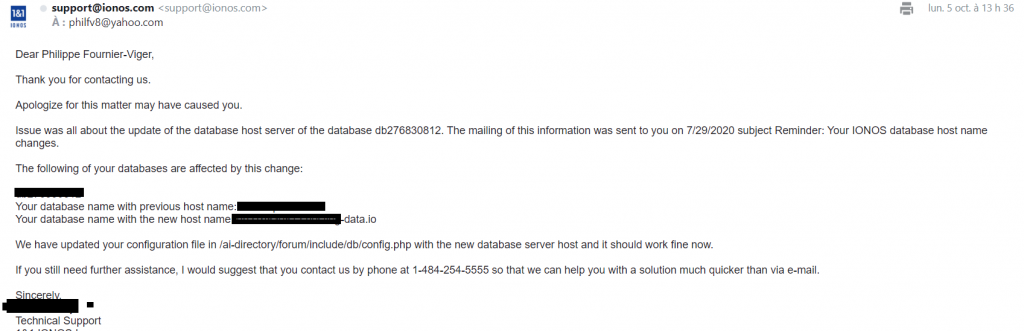
In that answer, 1&1 claims that the host name was wrong. However, I had updated it on October 1st to see if this was the problem. Moreover, I also tested conneting directly to the database through PHPMYADMIN from the 1&1 control panel and it was also down. So this was certainly not the problem. Thus, it seems that they do not want to admit that the problem was on their side and try to find some excuse about this to put the blame on the customer… This is similar to when my database was reverted to 3 years ago in August and I lost many blog posts on another website hosted on their service. They also did not want to say where the problem came from but it seemed to be obviously coming from them….
So, I am happy that the problem is fixed but I am still not very happy about their service. My data mining forum had been down for several days and when you pay for a hosting provider, you expect 99% availability, or at least a quick fix in case of technical problem from their side.