This year, I am attending the PAKDD 2019 conference (23rd Pacific Asia Conference on Knowledge Discovery and Data Mining), in Macau, China, from the 14th to the 17th April 2019. In this blog post, I will provide information about the conference.

About the PAKDD conference
PAKDD is one of the most important international conference on data mining, especially forAsia and the pacific area. I have attended this conference several times in recent years. I have written reports about the PAKDD 2014, PAKDD 2015, PAKDD 2017 and PAKDD 2018 conferences.
The proceedings of PAKDD are published in the Springer Lectures Notes on Artificial Intelligence (LNAI) series, which ensures good visibility for the paper. Until the end of May 2019, the proceedings of PAKDD 2019 can be downloaded for free.
This year, PAKDD 2019 received a record of 567 submissions from 46 countries. 25 papers were rejected because they did not follow the guidelines of the conference. Then, other papers were reviewed each by at least 3 reviewers. 137 papers have been accepted. Thus the acceptance rate is 24.1 %.
Location
The PAKDD conference was held at The Parisian hotel, a 5 stars hotel in Macau, China. Macau is a very nice city, located in the south of China. It has nice weather and some of its major industries are casinos and tourism. Macau was once occupied by Portugal before being returned to China. As a result, there is a certain Portuguese influence in Macau.
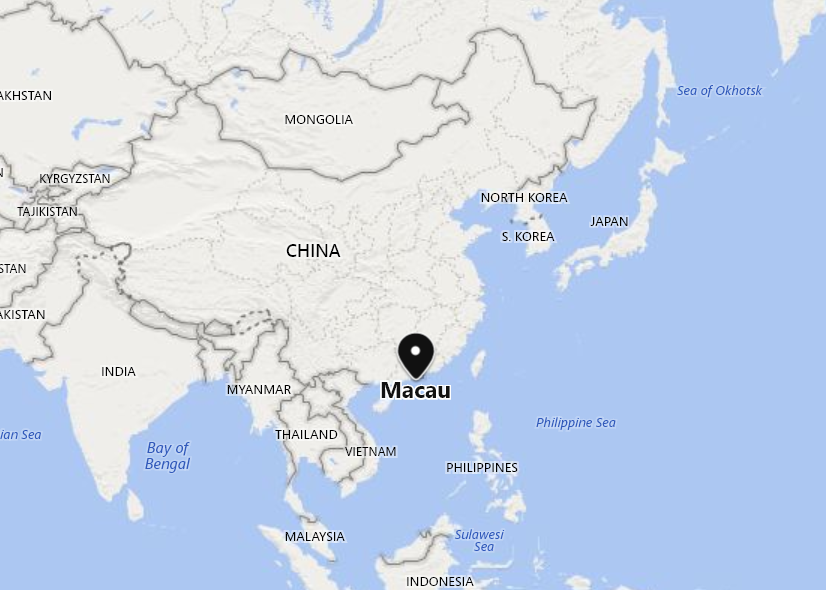
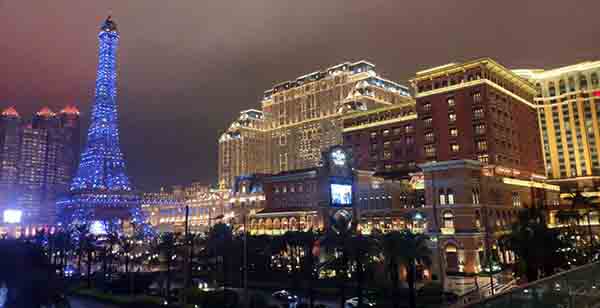
Day 0: Registration
On the first day, I arrived at the hotel and registered. The staff was very friendly. Below are some pictures of the registration area, the conference bags and materials. The bag is good-looking and contains the proceedings on a USB, the program, as well as some delicious local food as a gift.
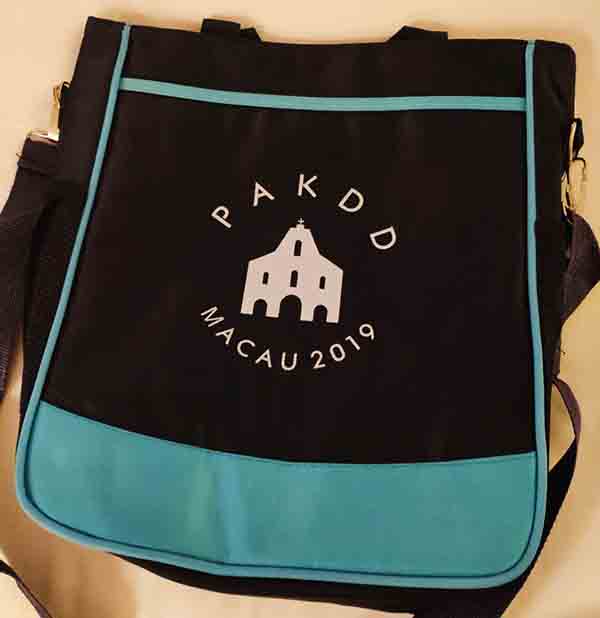
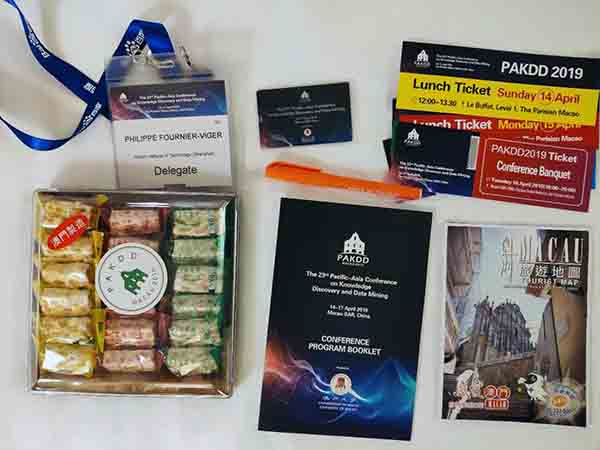

Day 1 : Tutorial: IoT BigData Stream Mining
In the morning, I have attended the IoT Big Data Stream Mining tutorial by Joao Gama, Albert Bifet, and Latifur Khan.
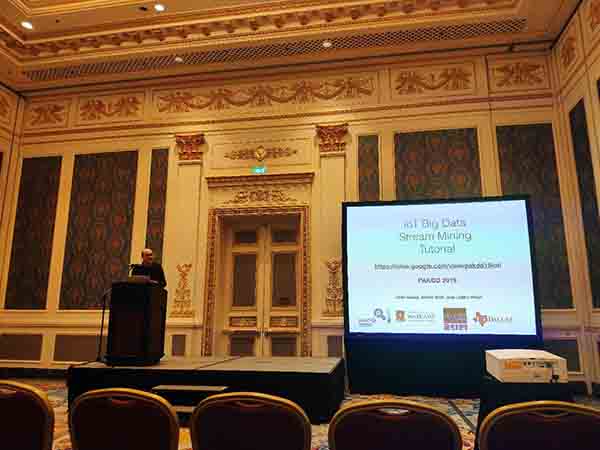
It was first discussed that IoT is a very important topic nowadays. According to Google Trends, IoT (Internet of Things) has became more popular than “Big Data”.
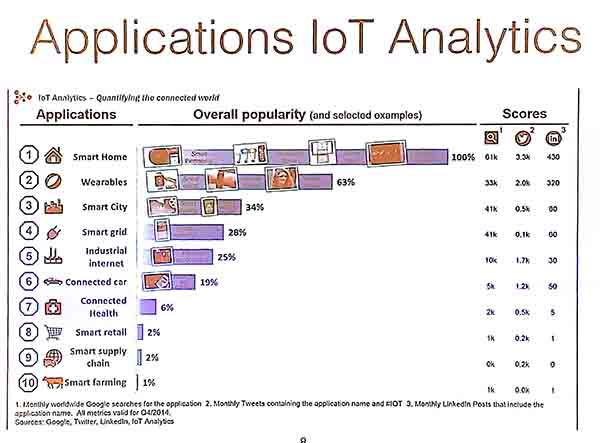
In traditional data mining, we often assume that we have a dataset to train a model. A key difference between traditional data mining and analyzing the data of IoT is that the data may not be a static dataset but a stream of data, coming from multiple devices. A data stream is a “continous flow of data generated at high-speed from a dynamic time-changing environment”. When dealing with a stream, we need to build a model that is updated in real-time and can fit in a limited amount of memory, to be able to do anytime predictions. Various tasks can be done on data streams such as classification, clustering, regression and pattern mining. Some key idea in stream mining is to extract summaries of the stream because all the data of a stream cannot be stored in memory. Then, the goal is to provide approximate predictions based on these summaries and provide an estimation of the error. It is also possible to not look at all the data but to take some data samples, and to estimate the error based on the sample size.
If you are interested in this topics, slides of this tutorial can be found here.
Day 1: Welcome reception
After the workshops and tutorials, there was a welcome reception in the evening at the Galaxy Hotel. There were drinks and food. It was a good opportunity for discussing with other researchers. I met several researchers that I knew and met several people that I did not knew.
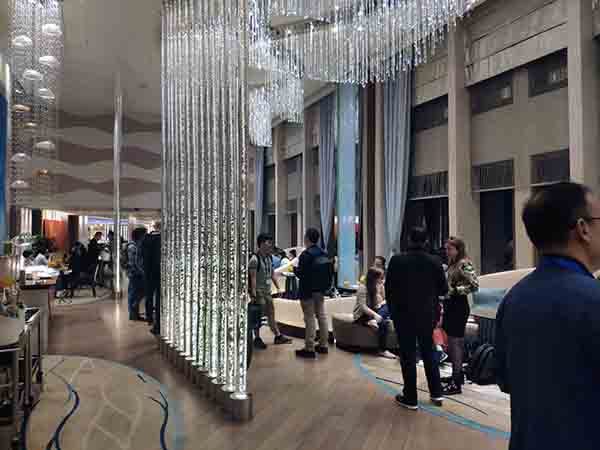
Day 2: Conference Opening
The second day started with the conference opening, where a traditional lion dance was first performed.
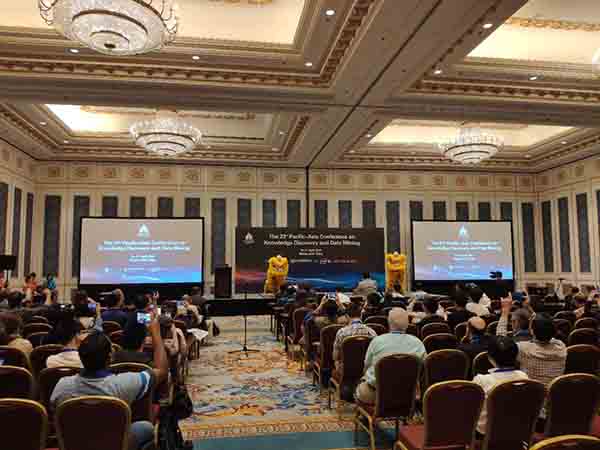
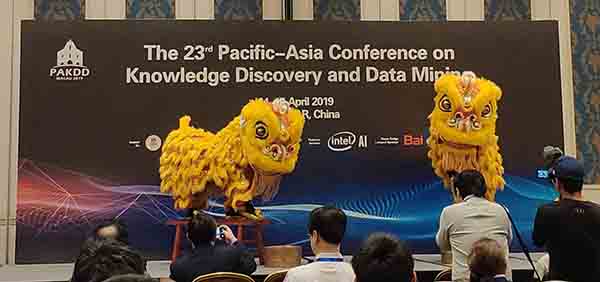
Then, the organizers talked. It was announced that there was more than 300 participants to the conference this year.

The PC chair gave information about the conference. Here are some pictures of some slides:
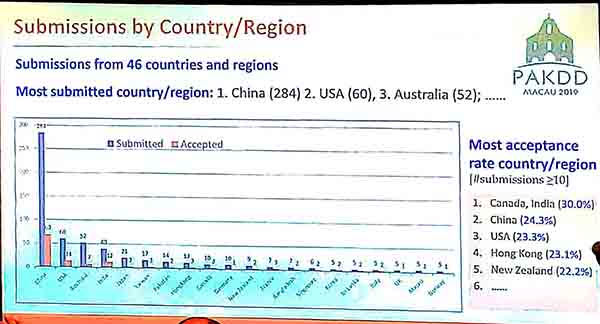

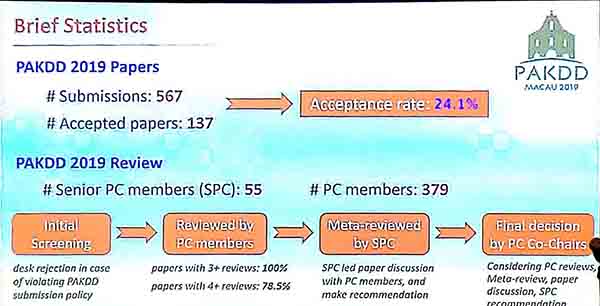
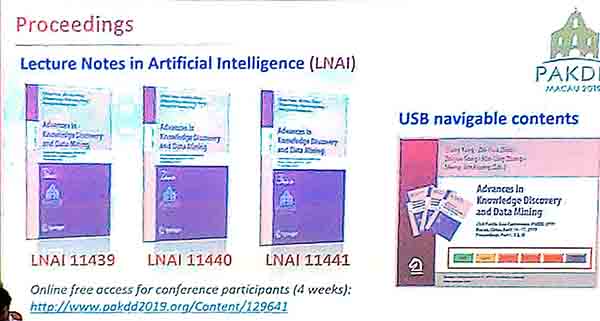
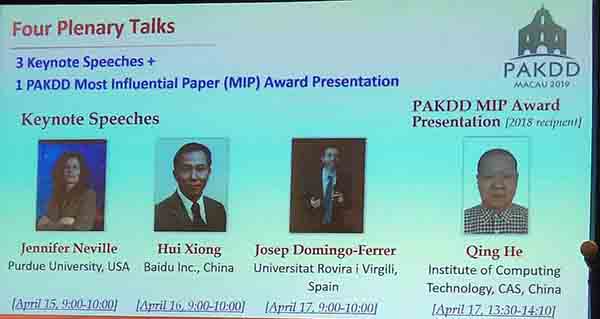

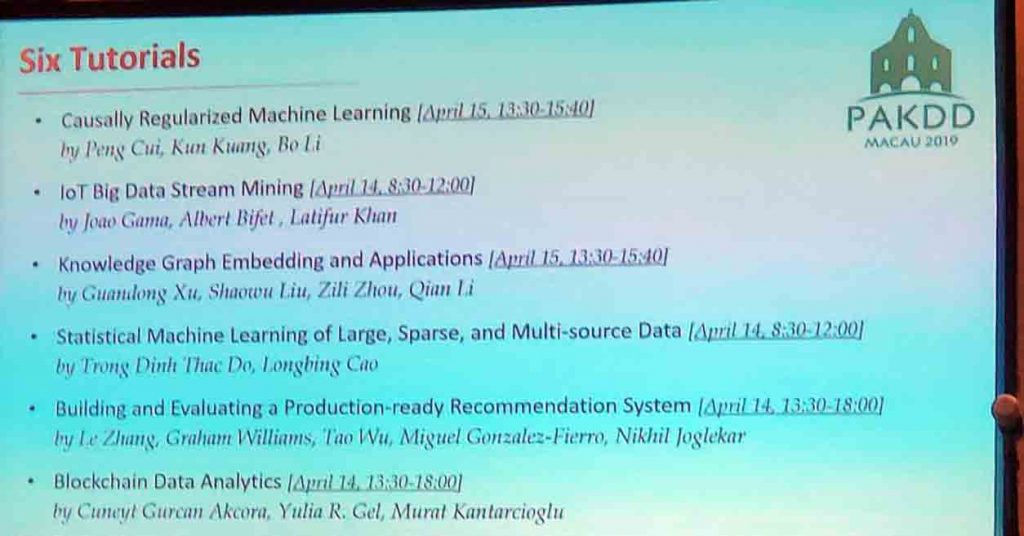

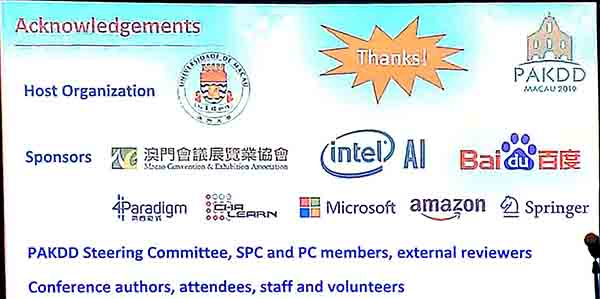
Then, there was a keynote about relational AI by Dr. Jennifer L. Neville. It was about the analysis of graph or networks such as social networks.

Then, there was several research paper presentations for the rest of the day. We presented a paper about high utility itemset mining called “Efficiently Finding High Utility-Frequent Itemsets using Cut off and Suffix Utility“.
In the evening, there were no activities were planned, so I went with other researcher to eat at a restaurant in the Taipa area.
Day 3: Keynote on Talent Analytics
In the morning, there was a keynote by prof. Hui Xiong about “Talent Analytics: Prospects and Opportunities”. The talk is about how to identify and manage talents, which is very important for companies.
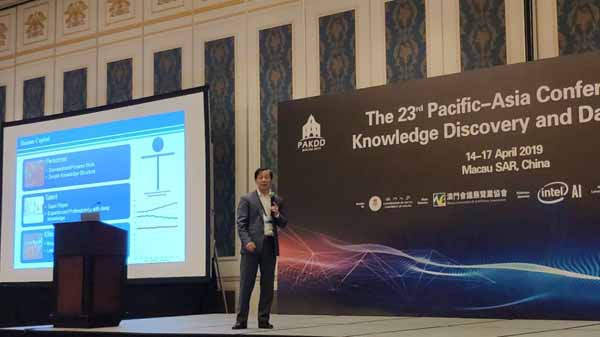
A talent is some “experienced professional with deep knowledge”. This is in contrast with personnel that do simple standardized work and have simple knowledge and may in the future be replaced by machines. Talents are team players and elite talents also have leadership. Leadership means to have vision about the current situation and what will happen in the next five years, be able to manage a team and manage risks. In terms of team management, it is important to find talents for the right positions and manage the team well.
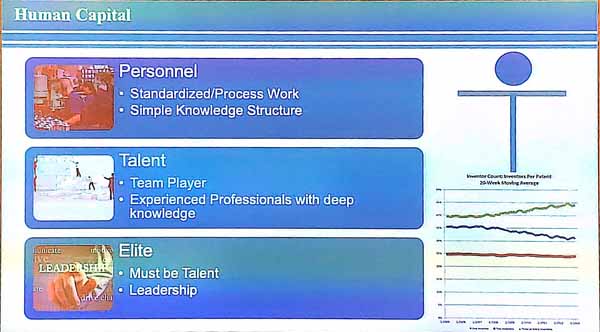

The presenter explained that intelligent talent management (ITM) means to use data with an objective, and to take decisions based on data, and to offer specific solution to complex scenarios and be able to do recommendations and predictions. Some examples of tasks are to predict when talents will leave, do intelligent recruitment, do intelligent talent development, management, organization, and risk control. Doing this well requires big data technical knowledge and human resource management knowledge.
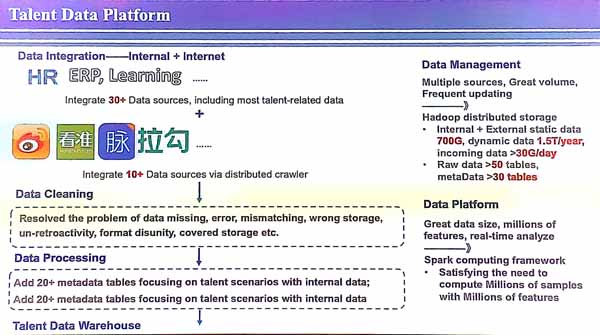
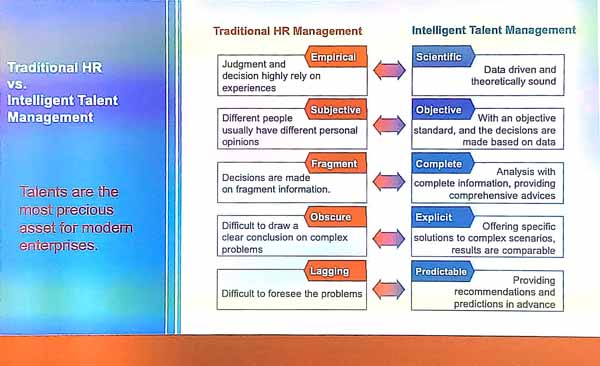
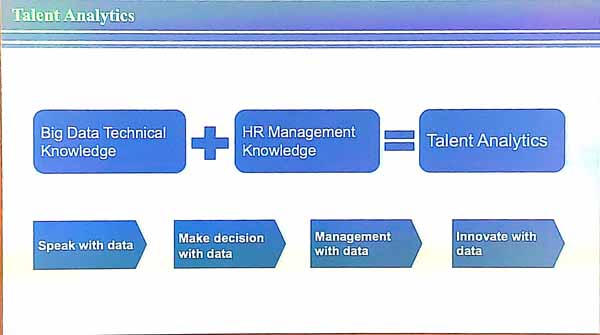
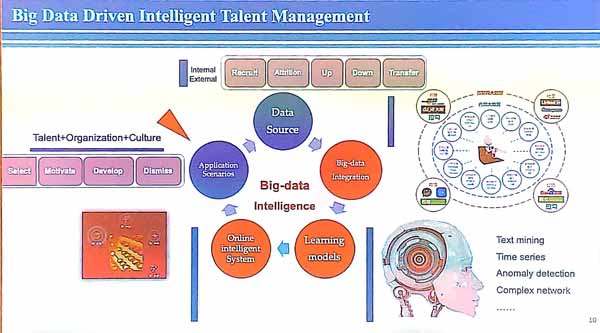
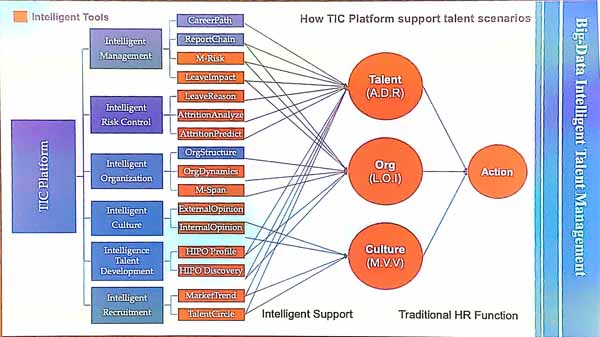
Then, there was paper presentations.
Day 3: Excursion and banquet
In the afternoon, there was a 4 hour city tour of St. Paul Ruin, Senado Square, A Ma temple and the Lotus flower square. Here are a few pictures.
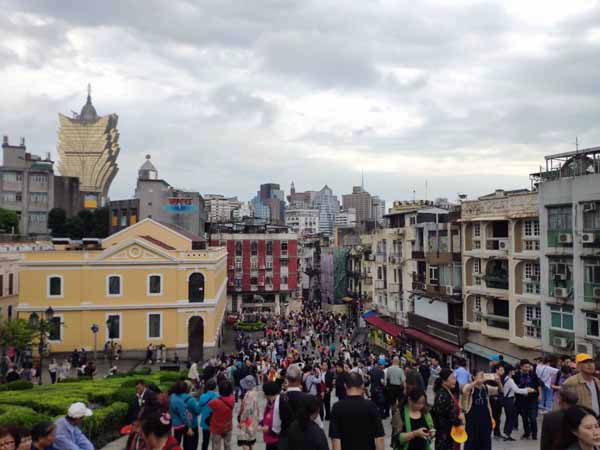

Finally, the conference banquet was held in the evening. Several awards were announced.

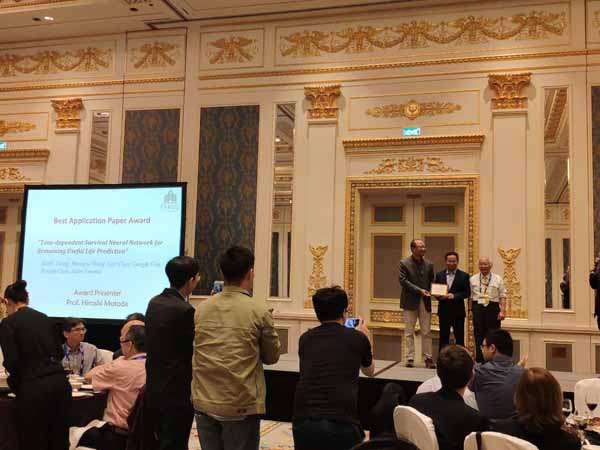
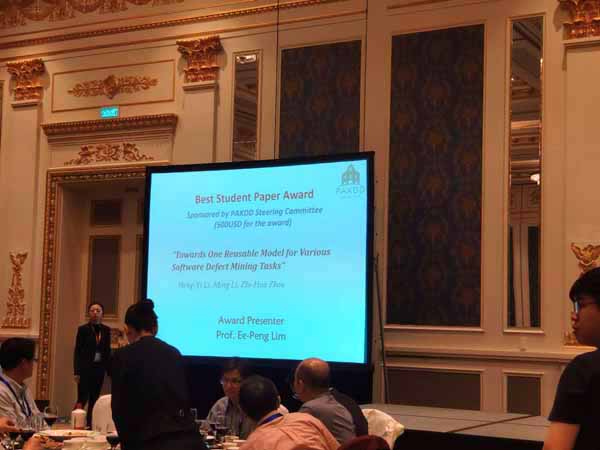
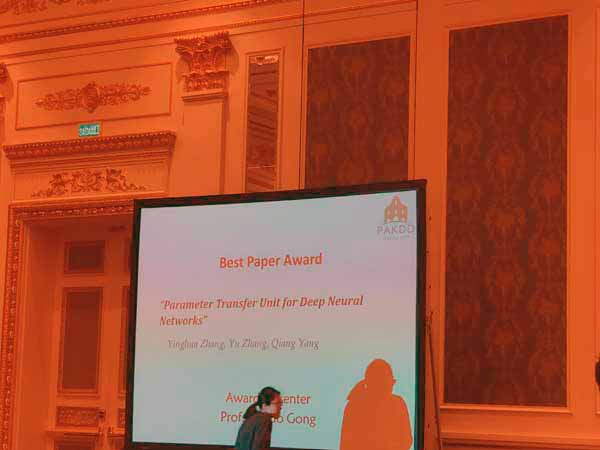
And there was some music and show during the banquet:
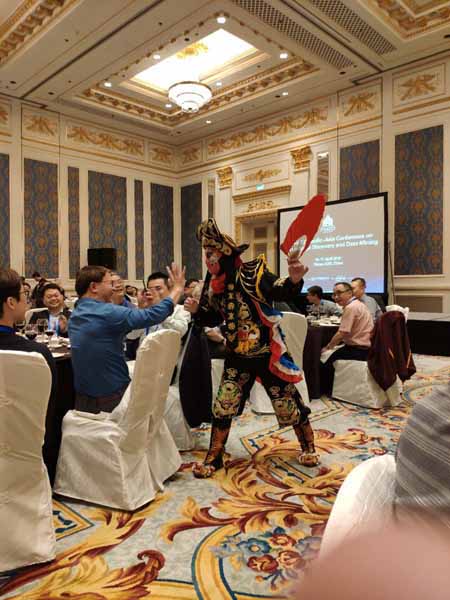
Day 4: Keynote Talk on Big Data Privacy
In the morning, there was a keynote talk by Josep Domingo-Ferrer about how to reconcile privacy with data analytics. He explained what is big data anonymization, limitation of the state of the art techniques, how to empower subjects, users and controllers, and opportunities for research.
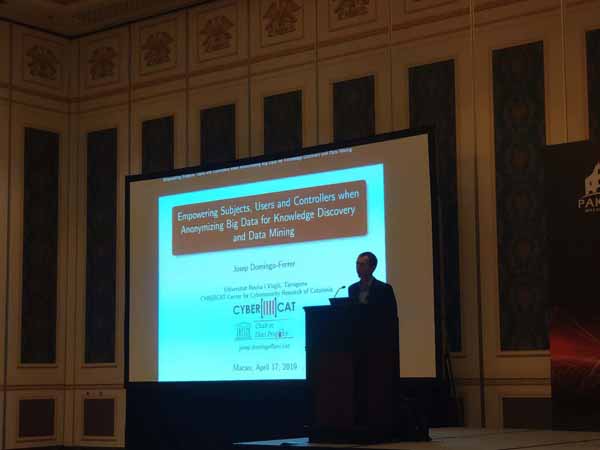
It was first discussed that several novels have anticipated the problem of data privacy, and nowadays many countries have adopted laws to protect data. A few principles are proposed to handle data: (1) only collect data that is needed that and keep it only as long as possible, (2) let the user give specific and explicit consent, and (3) limit collected data to some purpose, (4) the process should be open and transparent, (5) the ability to erase or rectify data, (6) protect data from security threats, (7) accountability, and (8) privacy should be in the design of the system.
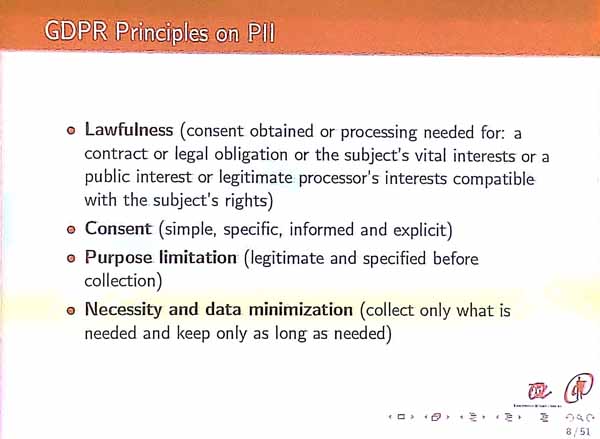
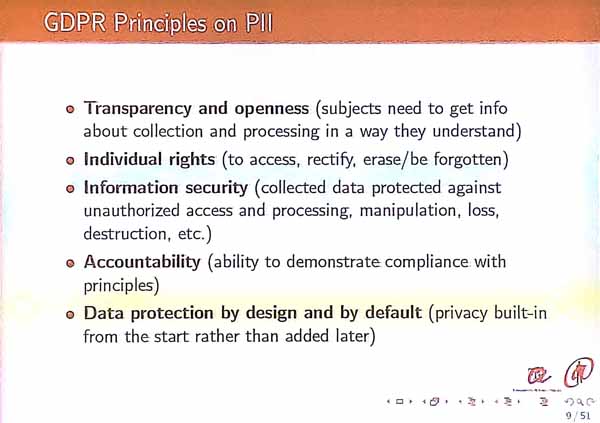
But it is sometimes complicated to comply with these principles. It seems to be in conflict with the use of big data.
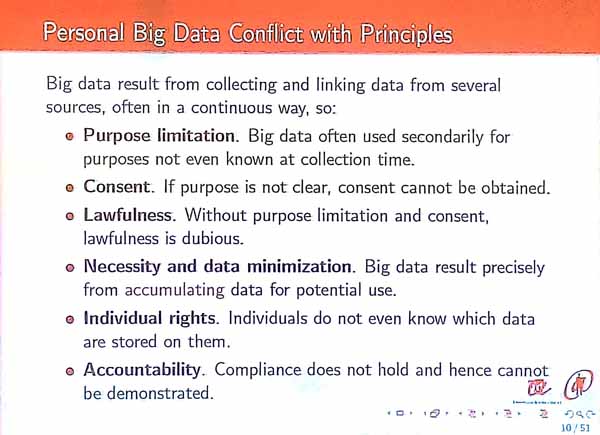
A solution is data anonymization. After we anonymize data, it may be easier to use the data for secondary uses. Thus a challenge is to create these anonymized big data sets.
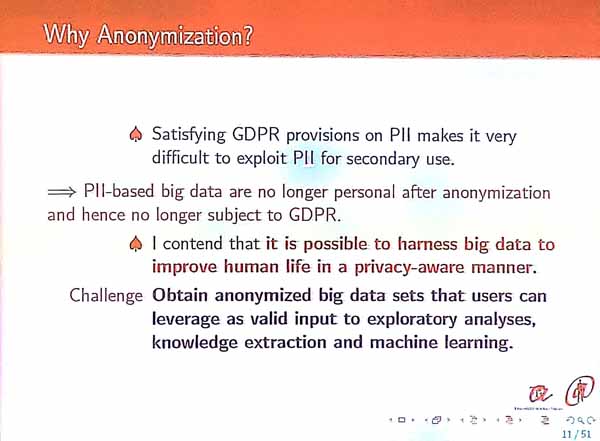
Statistical disclosure control is a set of techniques to anonymize data. It is used to reduce the risk that data is re-identified. A goal is often to anonymize the data to reduce the risks of disclosure while preserving the usefulness of the data (utility).
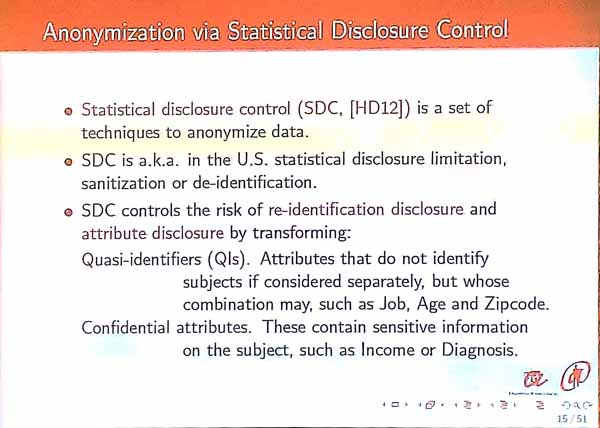

On the other hand, privacy-first models ensure that the anonymized data meet some minimum requirements. One of the most famous approach is called “k-anonymity“.
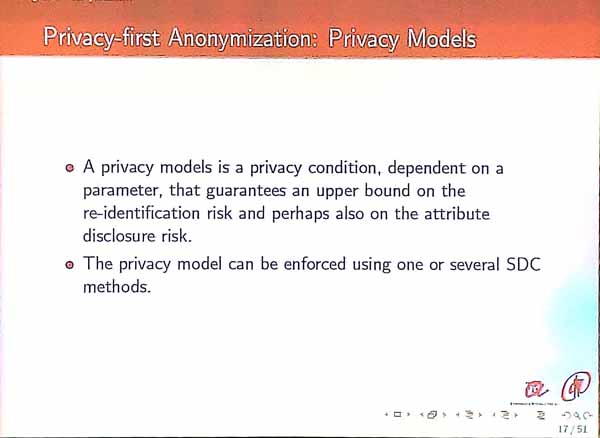
Other approaches are “differential privacy” techniques.
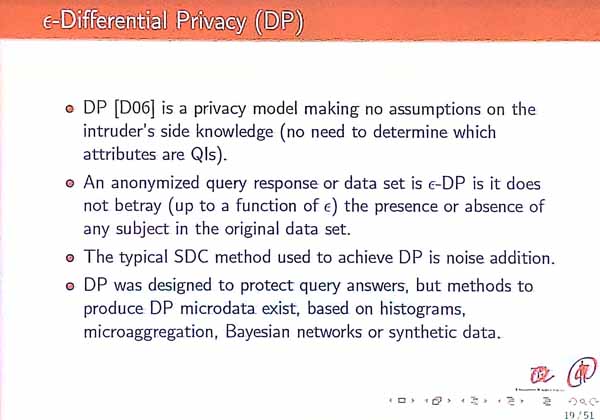
Some challenges related to privacy for big data is to ensure privacy in dynamic data (data streams). For big data, there are methods that anonymize data locally (e.g. by adding noise or generalization) before sending them to controller.
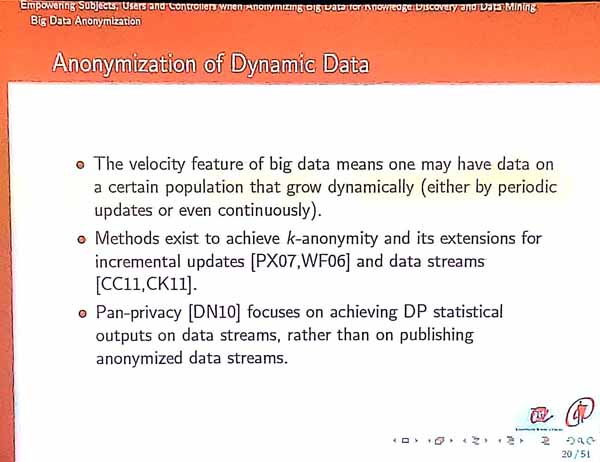
Some limitations of state-of-the-art techniques are as follows:
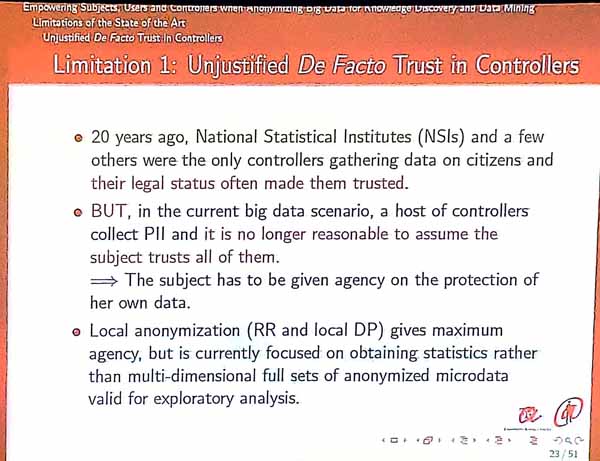
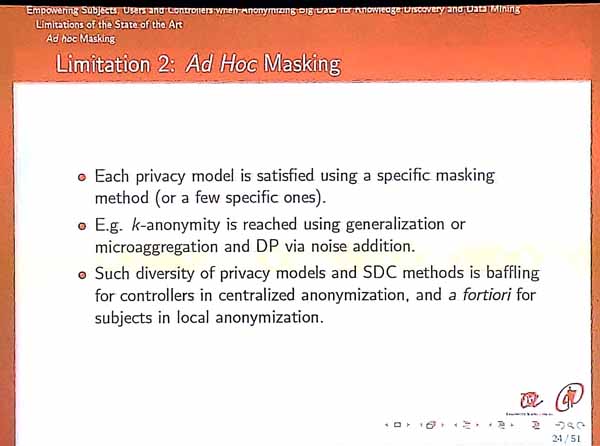
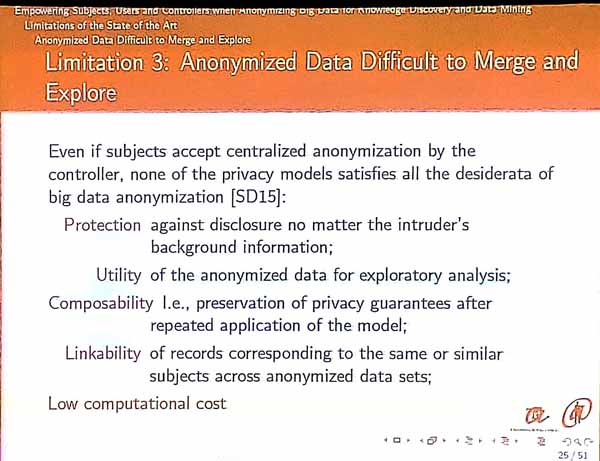
There was then some discussion of some proposals for privacy preserving big data analytics. I will not report all the details. The conclusions of the talk:
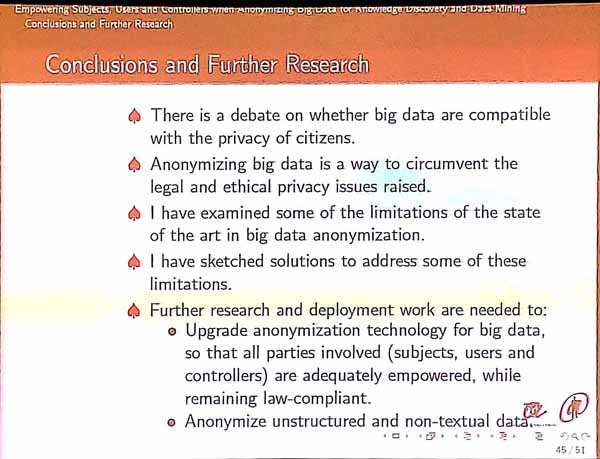
Day 4 – afternoon
In the afternoon, there was a PAKDD most influential paper award presentation on Extreme Support Vector Machine by Prof. Qing He, as well as the PAKDD 2019 Challenge Award presentation.
Conclusion
Overall, this was an excellent conference. It was well-organized. I met many researchers, listened to several interesting talks. Looking to PAKDD 2020 next year in Singapore.
Update: I have also written reports following this conference about PAKDD 2020 and PAKDD 2024.
—
Philippe Fournier-Viger is a computer science professor and founder of the SPMF open-source data mining library, which offers more than 170 algorithms for analyzing data, implemented in Java.
Pingback: The PAKDD 2015 Conference (a brief report) | The Data Blog
Pingback: The PAKDD 2017 conference (a brief report) | The Data Blog
Pingback: PAKDD 2018 Conference (a brief report) | The Data Blog
Pingback: The PAKDD 2020 conference (a brief report) | The Data Blog