There is a well known principle in statistics that correlation does not imply causation. It means that even if we observe that two variables behave in the same way, we should not conclude that the behavior of one of those variables is the cause (or is related) to the other.
In statistics and data mining, we can calculate the correlation between two variables or time series to see if they are correlated. The range of values for the correlation is usually [-1,1] where -1 indicates a negative correlation (two variables that behave in opposite ways, 0 indicates no correlation, and 1 indicates a positive correlation. Two variables that have a high correlation may be related. But if two variables have a high correlation but are not related, they are called a spurious correlations.
To be convinced of the principle that correlation does not imply causation, I will share a few examples from a very good website on this topic ( http://tylervigen.com/ ), that lists thousands of spurious correlations.

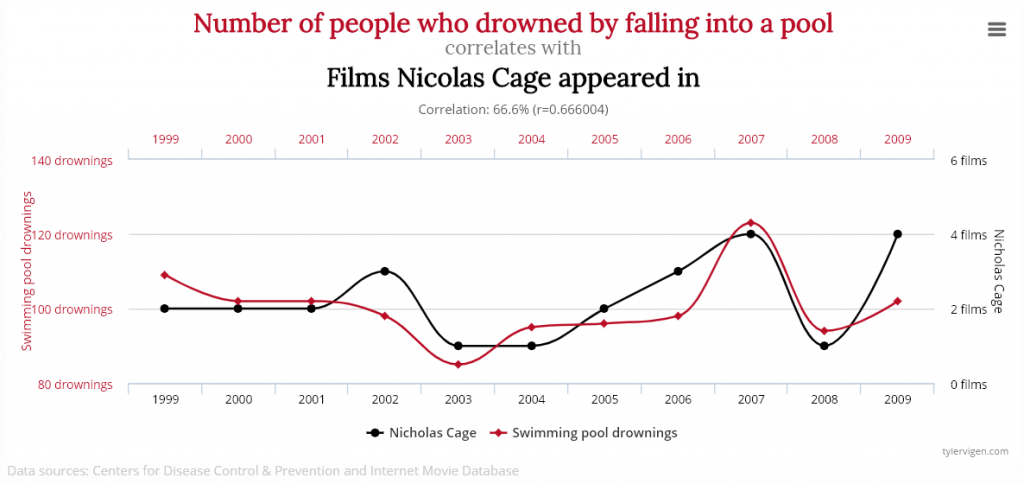
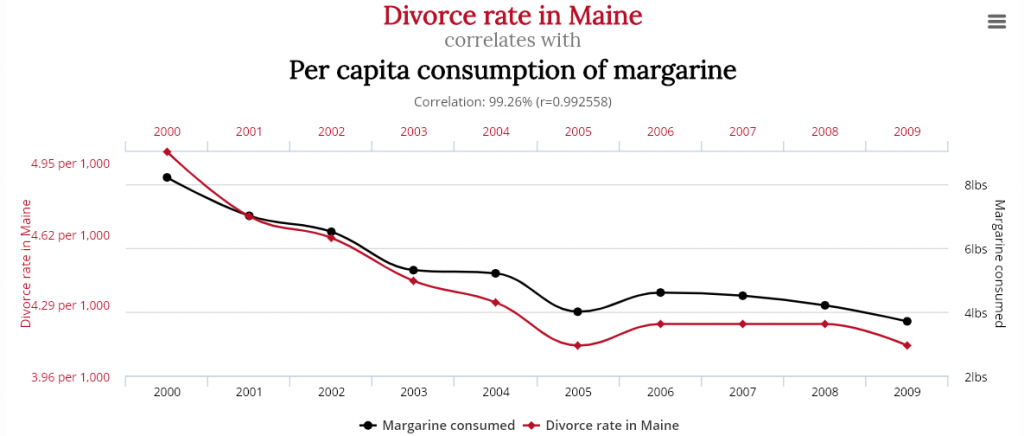
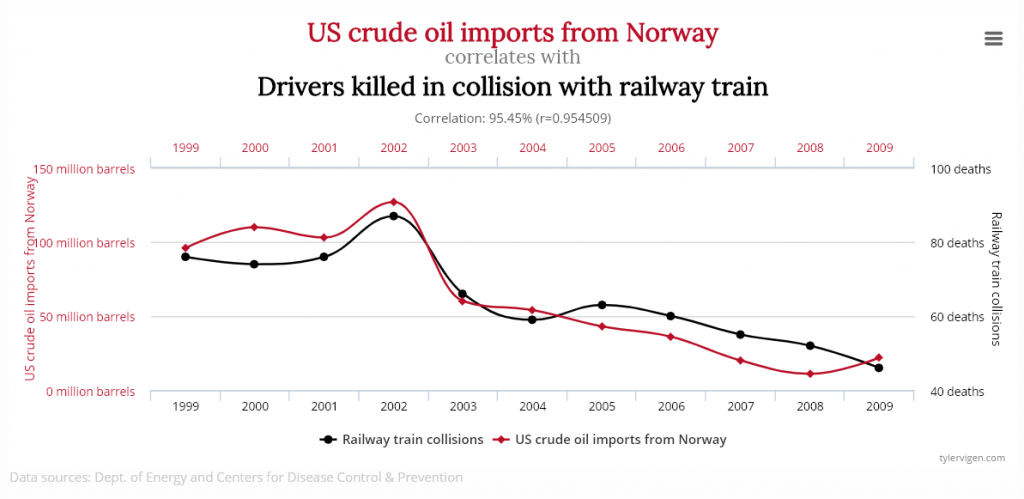
Obviously, these correlations are totally spurious although the variables show very similar behavior. This shows the needs to always look further than just using a correlation measure.
Those are just a few example of spurious correlations. If you try the website, you can also browse various variables to find other spurious correlations.
Conclusion
In this short blog post, I shown a few examples of spurious correlations at I think it is quite interesting. If you have comments, please share them in the comments section below.
—
Philippe Fournier-Viger is a full professor working in China and founder of the SPMF open source data mining software.